Abstract
Introduction. Environmentalists, governments, stakeholders, water resource planners and managers, and other concerned parties have turned to existing water quality indices and models to assess the condition of the world’s freshwater supplies. The introduction of heavy nutrient loads into waterways, stemming from agricultural runoff and urban sewage, disrupts the natural equilibrium of ecosystems. Monitoring programs often rely on in situ measurements to ensure the constant vigilance required for protecting human health and the natural world. However, traditional methods, involving the collection of water samples in nature and subsequent lab analysis, can be costly, time-consuming, and limited in addressing potential dangers comprehensively. Drought and water scarcity resulting from excessive heat can increase susceptibility to infections causing diarrhea. Waterborne diseases such as viral hepatitis, dysentery, typhoid, cholera, and more severe conditions like guinea worm can be contracted through drinking polluted water. The primary mode of transmission for waterborne diseases is the ingestion of contaminated water or food prepared with contaminated water. Water-washed disorders, including Shigella, skin illnesses, scabies, and trachoma affecting the eyes, are common.
The aim of this study is to mitigate and prevent water-related diseases through the implementation of effective water quality monitoring methods.
Material and methods. We present a real-time water quality monitoring approach based on linear regression. Various water quality characteristics, including but not limited to temperature, pH, ORP, EC, DO, and coliform bacteria, are measured and utilized in the monitoring process. This comprehensive monitoring strategy aims to contribute to the reduction of waterborne diseases.
Results. Were collected experimental evaluation data from water quality automated stations in the Hebei region to assess the effectiveness of a linear regression-based technique for predicting spatial and temporal correlations. The experimental data was gathered at the Langfang water quality Automated Station from July 25, 2018, to December 7, 2018. The dataset comprises a total of 13 variables, with 9 of them serving as indicators of water quality. These indicators include physical attributes like temperature, conductivity, and turbidity, as well as chemical water quality parameters such as pH, TN, TP, DO, CODMn, and NH3-N. Models were employed to predict the concentrations of TP and TN. A precision score exceeding 0.9 for both TP and TN indicates the robustness of the linear regression-based spatiotemporal connection forecasting approach, demonstrating high accuracy in predicting water quality.
Conclusions. Improper handling, such as touching water with unclean hands or using contaminated instruments to collect water for cooking or drinking, can lead to water pollution. This article maintains its focus on water–related illnesses, particularly in the context of the current global crisis stemming from a lack of access to clean water and sanitation. Real-time monitoring is deemed crucial in various areas, including environmental monitoring, lake and river water quality tracking, distribution network monitoring, and detecting pollution in drinking water. The proposed work can be adapted to various contexts, encompassing urban, rural, and environmental settings. Future research endeavors will prioritize integrating fuzzy logic-based water quality monitoring into existing infrastructure and networks.
|
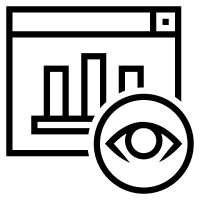
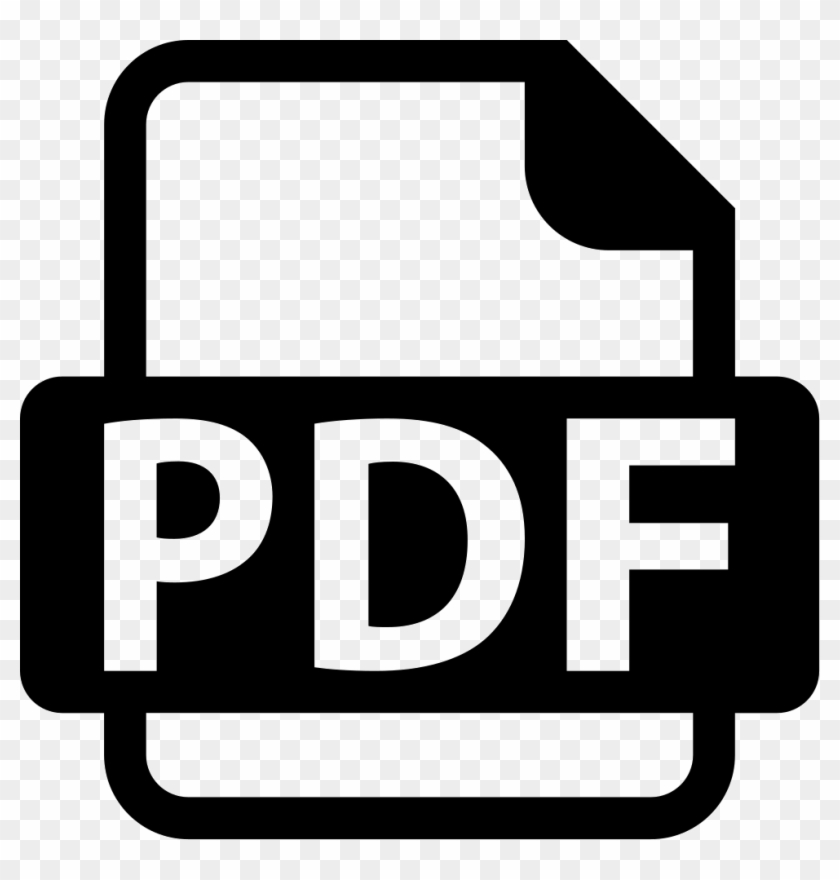

This work is licensed under a Creative Commons Attribution 4.0 International License.